We compare the ensemble mean against observations of sea-ice in addition to temperature and precipitation over land areas that enclose the Arctic basin. The predictions are evaluated separately for summer and winter. The skills of the decadal predictions are assessed by combining the results from all start dates and looking at the correlation and error as a function of the forecast length.
The goal of the COMBINE project is the improvement of climate models and simulations. For EC-EARTH we included an improved parameterization of the sea-ice albedo in the model version for Stream 2. The sea-ice initial conditions in Stream 1 (=contribution to CMIP5) originated from a forced NEMO run, for the Stream 2 experiments we used the new sea-ice re-analysis from UCL instead. The decadal simulations for CMIP5 were then repeated and the same evaluation performed.
Results from Stream2 show generally more skills than those from Stream1. However, neither Stream1 nor Stream2 score higher than climatology or persistence. A major problem in this evaluation is the small sample size that is the result of the small number of start dates. Currently SMHI is redoing the decadal predictions for CMIP5 with start dates every year in order to obtain better statistics.
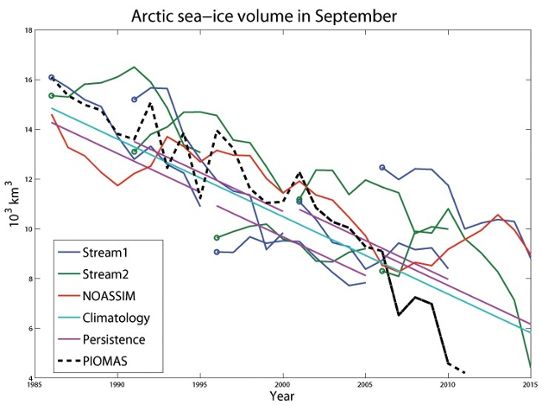
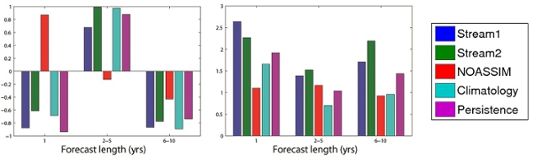