These pages present the development of the climate in Sweden up until 2018 and how climate may change during the 21st century. The service builds on observations and scenarios from several different regional climate models forced with several different global climate models instead of, as before, only one regional model. The horizontal resolution in the regional models has increased from 50 km to 12.5 km, which give more detailed results.
In climate model calculations, information on future changes in the atmosphere are used. Th climate models describe the connections between processes in the atmosphere, on the surface and in the oceans. The results of climate model simulations are refined for each of the counties in Sweden, and cover the period 1951-2100. The climate model simulations are a part of the international research programme CORDEX (cordex.org) and Copernicus Climate Change Service (climate.copernicus.eu).
As reference, maps based on a combination of observations and reanalyses are also presented from the period 1961-2018, compiled in SMHI:s reference data set. The reference data (SMHI Grid-Clim, an optimised combination of station data and the reanalysis UERRA) contain amongst others daily average temperature, daily maximum and minimum temperature and daily precipitation on a grid of 2,5 km resolution across Scandinavia.
Climate models
Climate models are used to calculate future climate. These are 3-dimensional representations of the atmosphere, the land surface, oceans, lakes and ice. In the model the atmosphere is divided into a 3-dimensional grid, across the surface and upwards. To get good results processes outside the entire atmosphere must be considered, that is around the globe and up in the air. Such models are called global climate models. The time evolution of different meteorological parameters are calculated in each cell of the grid.
Climate models create enormous amounts of information and requires therefore large amounts of computer power, which means that the 3-dimensional grid must be restrained. The grid in a global climate model is sparse, which gives less details on the regional scale. The grid is put on a smaller region in the regional model, for example Europe. In that way more detail can be achieved for a smaller region without using too much computer power.
The results from a global climate model governs what happens outside the model domain in the regional model. In that way the regional model also considers what happens outside the domain. In this service several regional climate models forced with several different global models are used. The regional models cover Europe and have a resolution (size of the grid boxes) of around 12.5x12.5 km.
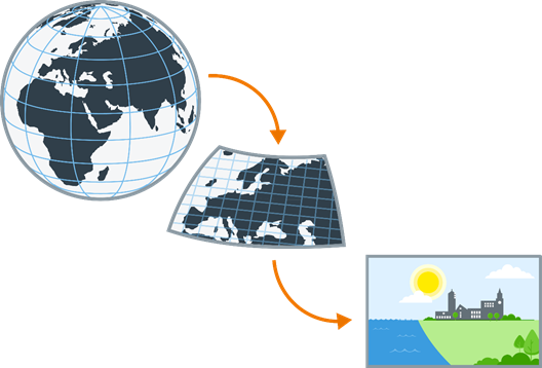
High resolution climate models require large resources, but more details can be achieved by so called downscaling. The higher resolution yields more data points which gives more details, climatological processes are also better described. This applies especially to short lived or local events. Precipitation in general and extreme precipitation in particular is an example of what can be improved by higher resolution. Precipitation may vary largely in space and time. That is better described by a model of high resolution (Rummukainen, 2010; Rummukainen, 2016).
Emissions Scenarios
Model simulations of the climate are based on emissions scenarios or radiative scenarios. Emissions scenarios are assumptions of future emissions of greenhouse gases. They are based on assumptions of future development of world economy, population growth, globalisation, transitions to environmentally friendly technology amongst others.
The amount of future emissions is affected by all these factors, which in turn affects the greenhouse effect. A measure of how the greenhouse effect is changing in the future is radiative forcing, measured in power per square metre (W/m2).
The more emissions of greenhouse gases the more radiative forcing. Such scenarios are called RCP scenarios (Representative Concentration Pathways (Moss et al., 2010; van Vuuren et al., 2011)).
In this analysis three scenarios are used:
- RCP2.6: Powerful climate policy makes emissions of greenhouse gases to culminate in year 2020, radiative forcing reaches 2.6 W/m² year 2100 (used in IPCC, AR5). This scenario is the one closest to the ambitions from the Paris Agreement.
- RCP4.5: Strategies for reduced greenhouse gas emissions yield a stabilisation of the radiative forcing at 4.5 W/m² before year 2100 (used in IPCC, AR5).
- RCP8.5: Increasing greenhouse gas emissions makes radiative forcing to reach 8.5 W/m² year 2100 (used in IPCC, AR5).
Climate scenarios
A climate scenario is a combination of emissions or radiative scenario, global climate model, regional climate model and a time period of choice. See the table in the section on ensembles for more information on the climate models used.
The regional models start somewhere between 1951 and 1971 depending on the model. It is possible that the results deviate from observations already in the beginning. This is because the global models used not exactly represent the climate of today in the same way in all simulations. The period 1971-2000 is used as reference. Results for the future shows anomalies compared to the average of 1971-2000.
Since the results are provided as a grid, so called gridded data, it can be difficult to directly compare model results and observations. Observations give the conditions at a specific point, whereas the models give the average for the entire grid cell. The description of observed climate in this service is based on gridded data and can thus more easily be compared to climate model data.
Reference data
In addition to data from regional climate models this service also includes maps based on a combination of observations and reanalysis for the period 1961-2018, the SMHI reference data set (SMHI GridClim). In the time plots these are used as a comparison to data from the climate models. The reference data set contains a gridded compilation of daily observations of daily average temperature, daily minimum and maximum temperature and daily precipitation. These climatic variables have been observed at SMHI weather stations over the entire country (more than 900 stations for temperature and more than 2 000 stations for precipitation). In addition to that observations from the neighbouring countries are included. A scrupulous quality control excludes physically unrealistic values.
Observed station data are compiled into a gridded data set to enable comparison with data from climate models. To be able to get data also from grid cells not containing any of the SMHI observation stations, a “first guess” from the regional reanalysis UERRA is used. The reanalysis is a 3D historical simulation of the atmosphere; at the surface and at higher levels. The advantage of a reanalysis as first guess is that the climatic variables get realistic values in areas without observations. The UERRA reanalysis is further downscaled from 11 km to 2.5 km horizontal resolution.
With aid from statistical methods the “first guess” is combined with station data. This is done to be able to deliver an optimal interpolation of the climatic variable at the surface, but also to get a higher spatial resolution (2.5 km); this reduces the deviation from observations and preserves the “pattern” from the reanalysis at the same time.
In the SMHI reference data set each parameter is represented by the average over a cell of 2.5 x 2.5 km; thus, it does not correspond exactly to the measured value at an observation point. The variability in time in station data will therefore be higher than the variability in the corresponding grid cell in the reference data set.
In the SMHI reference data set each parameter is represented by the average of a grid cell of 2.5 x 2.5 km; thus it is not exactly corresponding to the measured value at an observation point. The variability in time in the station data will therefore be higher than the variability for the corresponding grid cell in the reference data. As a by-product of the optimal interpolation method a confidence interval can be given around the grid cell average.
Summary: SMHI makes the judgement that SMHI GridClim gives a realistic interpolation of the climatic variables daily average temperature, daily maximum temperature, daily minimum temperature and daily precipitation at a grid covering Scandinavia.
- From this data set a number of climate indicators can be calculated.
- The same data set can also be used as a reference to calculate climate change for different parameters in climate projections (future climate).
- The data set can be used as reference to perform a bias adjustment of climate models (se section on bias adjustment).
Bias adjustment
Complex and intertwined processes in Earth’s climate system are not always straightforward to implement in climate models due to resolution, or simply by a lack of knowledge about some processes. The climate models will therefore inevitably introduce systematic deviations, or bias, of the modelled climate, in comparison to observations. Bias will differ between variables, regions of the world, and models. When comparing a historical and a future projection, moderate bias is mostly not causing issues to the assessment of climate change. However, bias can prevent an indicator from being reasonably described when it is based on absolute thresholds, such as frost days, tropical nights, or precipitation above a defined intensity. Further, impact models are often sensitive to bias when they are calibrated with observational data. For example, the storage of water in frozen form as ice and snow in hydrological models requires a good description of temperature.
To prevent bias from generating misleading results in threshold based indicators, a so-called bias adjustment is first applied to the data. The data in the service has been bias adjusted using the MIdAS method (MultI-scale bias AdjuStment; Berg et al., SMHI Klimatologirapport nr 63). MIdAS is developed at SMHI and has been shown to perform well, also compared to international state-of-the-art methods. First, the method derives a mapping of values from the biased model to the reference data set. Remapping is then performed across the complete timeseries of the models, across the full distribution of values, and also for periods outside the reference period, such as for future projections.
Scenarios are not forecasts
The results from calculations made with climate models presented here are called climate scenarios, they are not weather forecasts. Climate scenarios are based on assumptions of future emissions and amounts of greenhouse gases. They represent the statistical behaviour of the weather of a longer period of time - that is the climate.
Indicators
In addition to temperature and precipitation a number of so-called climate indicators are calculated. Indicators are calculated from ordinary meteorological parameters. It may be the number of cold or hot days, accumulated precipitation of a week or the length of the vegetation period. Since many indicators are based on thresholds (for example when temperature exceeds a certain limit) they are sensitive to systematic deviations in the climate models. For example, if a model is a bit too cold it can have a large impact on the number of hot days. The calculations of indicators are thus based on bias adjusted data (see section on bias adjustment).
Name | Definition | Unit |
---|---|---|
Temperature | Daily average temperature | °C |
Maximum temperature | Daily maximum temperature | °C |
Minimum temperature | Daily minimum temperature | °C |
Precipitation | Average precipitation | mm/mon |
Diurnal temperature range | Maximum temperature minus minimum temperature | °C |
Cooling degree days | Degree days with average temperature > 20 °C | Degree days |
Heating degree days | Degree days with average temperature < 17 °C | Degree days |
Zero crossings | Number of days with maximum temperature > 0 °C and minimum temperature < 0 °C | Number of days |
Frost days | Number of days with minimum temperature < 0 ºC | Number of days |
Days with heavy precipitation | Number of days with precipitation > 10 mm/day | Number of days |
Days with extreme precipitation | Number of days with precipitation > 25 mm/day | Number of days |
Dry days | Number of days with precipitation < 1 mm | Number of days |
Longest dry period | Longest consecutive period with precipitation < 1 mm/day | Number of days |
Start of vegetation period | First day of a consecutive six-day period with daily average temperature > 5 °C | Day number |
Length of vegetation period | The start of the vegetation period is the first day of a consecutive six-day period with daily average temperature > 5 °C. The end of the vegetation period is the day before the first consecutive six-day period after 1 July with daily average temperature < 5 °C. The length of the vegetation period is the number of days from the first day until and including the last day of the vegetation period. | Number of days |
Summer days | Number of days with maximum temperature > 25 °C | Number of days |
Longest period of summer days | Longest consecutive period with summer days (maximum temperature > 25 °C) | Number of days |
Tropical days | Number of days with minimum temperature > 20 °C | Number of days |
Cold days | Number of days with maximum temperature < -7 °C | Number of days |
Why are different reference periods used?
The present climate is described by the last finished 30-year normal period, 1991-2020. Previous climates are described by earlier normal periods, and for the study of climate change different normal periods are compared.
To study the ongoing climate change the reference normal period 1961-1990 is usually used. In some cases it might be relevant to use other normal periods, for example when pre-industrial time is used as a reference. Within climate science other periods are sometimes used. In the beginning of the 21st century the period 1961-1990 was not seen as representative for the current climate, and it was still too early to shift to 1991-2020. Because of this IPCC has, for example, sometimes used the last 20-30 years as the reference to which the calculated climate change is compared with. Sometimes there are problems with choosing a period too close to the present.
In many climate simulations the greenhouse gas emissions scenarios start already in 2006, which means that the future starts in 2006 and that the years from then on do not represent actual levels of greenhouse gases. There may also be practical reasons not to use 1961-1990.
Climate model simulations require large computer resources. Therefore, it sometimes happens, that simulations start after 1961 to save computer power. As a compromise the period 1971-2000 is then used as a compromise between computer power and historical reference.
Read more about reference periods (in Swedish)
Calculations for the end of the century
The end of the century period is defined as the years 2071-2100. A few climate models lack data for the last years of that period. Formally, they can therefore not be used to derive information for that period. However, the lack of a small part of the 30-year period makes very little difference to the end result. Therefore, the service uses a condition that at least 25 years must be available in the period to allow the model to be used in the calculations, and still be presented as valid for the period 2071-2100. This approach is chosen because of the very few models that lack data, and the insignificant impact on the end result.
About ensembles
What is an ensemble?
An ensemble is a collection of climate model scenarios, or ensemble members. The members in the ensemble can differ regarding the driving global climate model, the regional climate model, or the emission scenario.
Why should one use model ensembles?
An ensemble provides an overview of the spread in the future climate scenarios. The spread informs about uncertainty in the results, which is important for assessing the robustness and reliability of the information. The reliability of the result increases if several models produce a consistent result.
The role of the climate model
The climate models, both global and regional, will provide different responses to the forcing from a given emissions scenario. These differences arise due to the models’ approach to simulate different physical processes in the atmosphere, which relate to our incomplete understanding of certain processes and the ability to numerically simulate them.
The global climate models determine the main climate sensitivity, and the large-scale atmospheric circulation. They will therefore have the largest impact on the simulated large-scale climate, such as wintertime mean temperature. Other processes are determined at smaller scales, such as summertime precipitation which arise from local cloud processes, where the regional climate models to a large extent dictate the results (Kjellström et al., 2018; Sørland et al., 2018).
It is challenging to select a single best performing climate model, because the models differ in performance depending on the region, the variable and season studied. Including several models acts to increase the robustness and quality of the ensemble mean result, and the most common approach is to include as many models as possible in an ensemble.
Another kind of ensemble can be configured by making several simulations with a single global climate model, where the initial conditions at the start-up of the model leads to different results. This is an effect of the chaotic development of the atmosphere, which in itself leads to a spread in the results, which is related to the natural variability of the atmosphere.
Ensembles describe the reliability of the results
The spread within the ensemble provides information on the reliability of the results. The set up of the ensemble can describe different uncertainties, such as model uncertainty or natural variability.
This service presents two measures of robustness and model spread: the relative number of models that show and increase, and the standard deviation across the model ensemble.
The relative number of models that show and increase, presents the model agreement on the sign of the change. If most models agree on an increase, the result can be considered robust. Further, if most models disagree on an increase, it means that they agree on a decrease. If the models show little agreement on the sign of the change, the result is either not robust, or there is no significant change to be expected from the studied variable. The latter requires further investigations.
Standard deviation is a measure of the model spread. Even a robust signal can have significant spread when the magnitude of the change is uncertain. A large spread results in a large value of the standard deviation relative to the magnitude of the change.
The time period matters
The number of models and scenarios to include in an ensemble partly depends on the time period studied. Generally, for the near future (a few decades into the future), the initial conditions influence the results and demands a large ensemble of different model and different initial states. At longer time horizons, the emission scenarios become more important than the initial conditions, but also the sensitivity of the climate model to the greenhouse gas forcing plays a large role.
Natural variability is important in the short term perspective
The climate system has it’s own natural variability in addition to anthropogenic effects. These natural fluctuations between years, or between decades complicates the analysis of calculated climate scenarios. Especially when studying climate change in the near term future. Climate change until year 2100 is calculated to be big enough to be clearly visible even with large variations between years. The natural variations of the climate can not be exactly predicted (for example for a certain date). However, it is possible to study natural variability by creating an ensemble of several climate scenarios based on one emissions scenario, but with different initial conditions. By the end of the century the largest uncertainty comes from the choice of climate model and the choice of emissions scenario.
Models used in this service
The principle is to use as many model simulations as possible, since more data give a better statistical foundation. It is also desirable with ensembles that do not lean too much on specific models. In that case certain models get a disproportionate significance. It may also be desirable that the RCP ensembles is of comparable sizes. The supply of simulations of RCP8.5 is significantly larger than for the other RCPs. There are also model combinations that have only been run for one or two RCPs. Since we want as big ensembles as possible, we will have to accept that the ensembles are not containing exactly the same models. Global models used by only a small number of RCMs and for only one RCP are omitted so that they do not contribute to the skewness between the ensembles.
Robustness of the results
Robustness is here a measure of how certain or uncertain the climate change signal is. Since the results are based on several different simulations with climate models it is possible to do statistical analyses of how robust (i.e. reliant) the results are. Here, two measures of robustness are used: the relative number of models that give an increase and the standard deviation.
The relative number of models that give an increase is a measure of how large part of the ensemble that agrees on a positive change. The greater the share of models that point in the same direction, the more robust the result. If all models in the ensemble give a temperature increase that is, for example, a robust result. It is also a robust result if no models give an increase. The result is not robust if, on the other hand, the models are relatively equally spread between increase and decrease. One example is shown in the left figure below. Dark green colours mean that most models agree on an increase. Outside the coast of Norway, for example, the the relative number of models that give an increase is 100 %; a robust signal. Purple colours means that most models do not give an increase. In parts of Finland no models give an increase (0 % give increase); that is also a robust signal.
Another measure of the robustness is the standard deviation. It shows how large the spread is between the models. The standard deviation is large when the spread is large, and small when the spread is small. The value of one standard deviation means that ca 68 % of the models are within that value. The spread is relatively small in southern Sweden and northern Finland in the example to the right below. Certain grid cells deviate from the general pattern. That usually means that one, or a couple of, models deviates from most of the others, for example by having a strong climate change signal. That can be due to differences in how the grid cell is characterised in different models. A grid cell can in one model be covered by water and in another covered by land. This effect is most obvious along coast lines and regions rich of lakes.
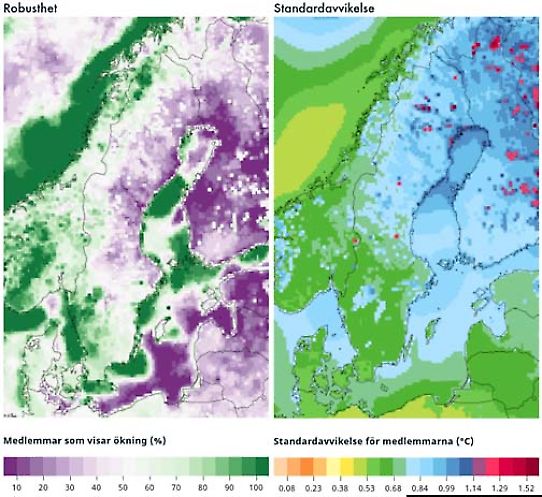
Updates
Here, there is room for descriptions of updates made.